Political Scientists often test their hypotheses using quantitative methods. When new methods are proposed, researchers are interested in how they perform relative to existing methods, and typically compare them using Monte Carlo experiments to compute performance statistics, such as bias. Although the discipline has made great strides in proposing and assessing new methods using Monte Carlo analyses, the advice from such developments has been based on too little information. Our literature review demonstrates that researchers largely report only one or two performance statistics, typically bias and root mean squared error (RMSE).
We argue that researchers can make more informed decisions through a comprehensive framework in which they also report and combine standard deviation (SD), overconfidence, coverage, and power. We offer a structured way to think about what gets reported, what might be missing, and how this should influence decisions about which method to use. Combining these six performance statistics can help researchers design their Monte Carlo experiments and help readers think critically about results.
We define each performance statistic as follows:
- Bias is the difference between the expected value of an estimated quantity from
repeated sampling and the value of that quantity in the data-generating process
(DGP). - SD is the square root of the variance of estimates
- Overconfidence is the standard deviation of the estimates divided by the expected
value of the estimated standard errors for a quantity of interest. - RMSE is the square root of the expected value of the squared differences between
the estimates of the quantity and the value of the quantity in the DGP from repeated
sampling. - Coverage is the proportion of times the confidence intervals of an estimate encompass the true value in the DGP.
- Power is the proportion of instances in which a null hypothesis is correctly rejected
from repeated sampling and estimation.
We also visualize each statistic. For example, imagine we conducted hypothetical simulations for an estimator, ˆθ, of the true parameter value θ. The gray bars in Figure 1 depict the density of the estimated values of θ, and the black vertical line in the center of the figure indicates the expected or average value of ˆθ. The dotted line on the left side shows the value of the “false” null hypothesis, specified as zero, while the one on the right side shows the true value. Under the histogram, we summarize eight of these hypothetical simulations. For each, we show the point estimate with a 95% confidence interval. We measure coverage as the proportion of intervals that contain the true value and power as the proportion that do not contain the null hypothesis. As shown in Figure 1, RMSE is the square root of the expected value of the squared differences between the estimates and the true value. Since RMSE is the combination of bias and standard deviation, lower values are generally preferred.
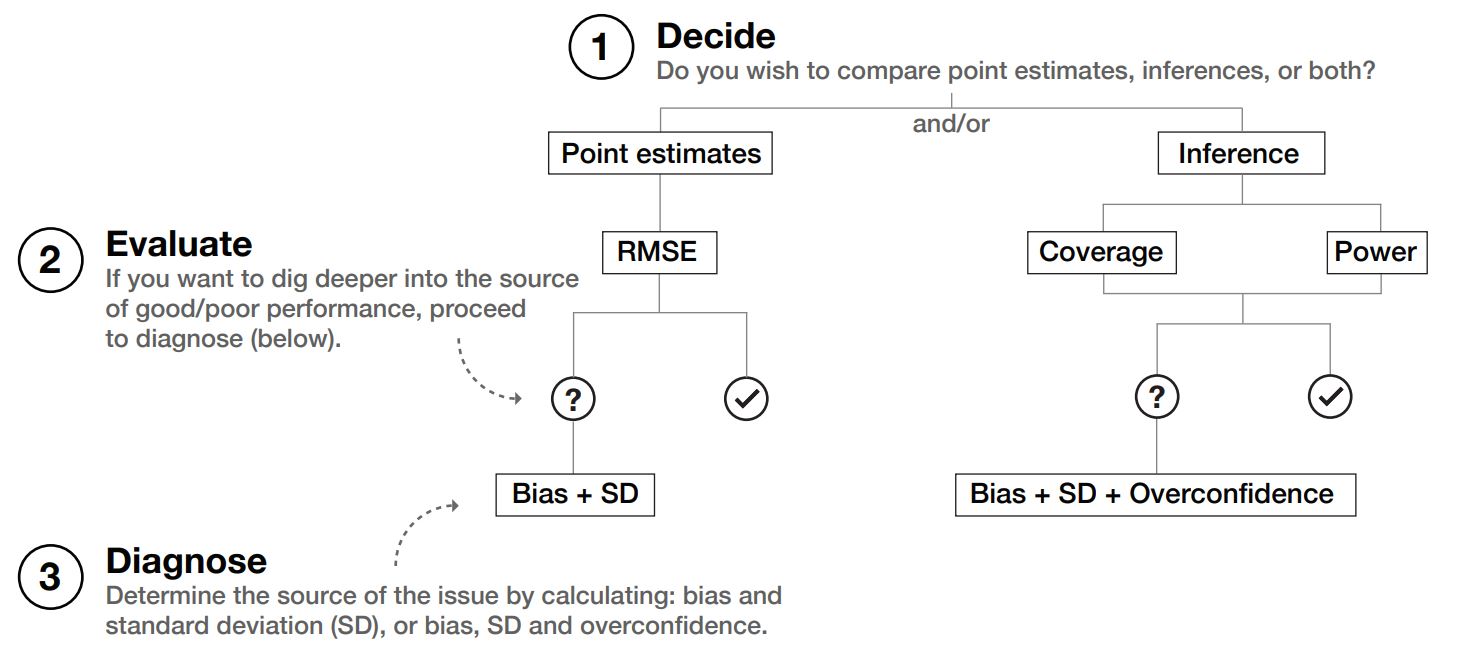
In Figure 2, we classify estimator performance in terms of point estimates and inference and expect both producers and readers of Monte Carlo experiments to be interested in each of these properties. It is also important to think about the value of the different performance statistics in combination with each other. The first group of performance statistics—RMSE and coverage probability and power—evaluates an estimator’s performance on point estimates and inference. The second group of performance statistics—bias, SD, and overconfidence—helps to diagnose why an estimator has large or small average error (RMSE), high or low coverage probability, and/or power. We recommend researchers begin by using the first group of performance statistics to evaluate how an esestimator performs in terms of point estimates and inference, and then, if needed, diagnose and understand these results using the second group of performance statistics.
Evaluation: RMSE summarizes how to point estimates differ from the true parameter value due to the systematic over/under-estimation of an estimator (bias) and the sampling variability (SD). It thus summarizes overall how far off the estimate will be, on average, from the true value. Coverage probability and power inform researchers whether Type 1 and Type 2 errors will be inflated, respectively.
Diagnosis: A small RMSE informs us that both bias and SD are small. But, a large RMSE could be due to large bias, large SD, or both. Two estimators may have similar RMSE but different levels of bias and SD. Diagnosing large RMSE means examining bias and SD in combination. Similarly, coverage and power are functions of bias, SD, and overconfidence, or some combination of the three. Consider an estimator that correctly estimates standard errors but exhibits bias. Our point estimate might be far off from the true parameter value, but if the uncertainty is large, then this value could still fall within the confidence interval. Similarly, smaller SD and/or overconfidence should increase power, but the latter does so by incorrectly estimating the precision of the estimate. Consider a scenario in which there is bias (e.g., the coefficient underestimates the true value) and overconfidence. In this case, power will be artificially greater than when bias is absent. Overall, in order to diagnose the source of problems of inference due to poor power and/or coverage probability, we recommend examining bias, overconfidence, and SD in combination.
We apply our framework—evaluation and diagnosis—to three replications. In two cases, we reach conclusions that substantially differ from those of the original authors; in other words, when taking into account all performance statistics, we prefer a different estimator than that suggested by the authors. In the third paper, we reach a similar conclusion as the original authors and demonstrate that their recommended approach is robust to our battery of performance statistics.
We urge researchers to consider our approach. Combining performance statistics can help producers structure their Monte Carlo experiments and help readers think critically and systematically about the results from these experiments.
Notes
Fig. 1: Illustration of RMSE, coverage, and power
This blog piece is based on a forthcoming article “How Do We Know What We Know? Learning from Monte Carlo Simulations” by Vincent Hopkins, Ali Kagalwala, Andrew Q. Philips, Mark Pickup, and Guy D. Whitten.
The empirical analysis has been successfully replicated by the JOP and the replication files are available in the JOP Dataverse.
About the Authors
Vince Hopkins is an Assistant Professor of Political Science at the University of British Columbia. He specializes in Canadian Politics, Behavioural Science, and Public Policy. He focuses on improving access to public services, using a combination of field/survey experiments, interviews, and participatory co-design. His work appears in venues such as Political Science Research and Methods, Political Research Quarterly, Policy Studies Journal, and Journal of Policy Analysis and Management.
Ali Kagalwala is a Visiting Assistant Professor in the Department of Political Science in the Bush School of Government and Public Service at Texas A&M University. His primary research interests include political economy, polarization, time series analysis, spatial econometrics, panel data, sample selection, and machine learning. His research has appeared in journals such as the American Political Science Review and The Stata Journal.
Andrew Q. Philips is an associate professor in the Department of Political Science, University of Colorado Boulder, and a research fellow at the University of Colorado’s Institute of Behavioral Science. He received his PhD from Texas A&M University in 2017. Philips has published over two dozen articles on topics such as political economy, comparative politics, and gender and politics, and is the author of a 2023 Cambridge University Press book on political budgeting. His research agenda also has a large methodological component, with interests in machine learning, time series, panel and compositional data.
Mark Pickup is a Professor of Political Science at Simon Fraser University. He is a specialist in Political Behaviour, Political Psychology and Political Methodology. Substantively, his research primarily falls into three areas: political identities and political decision-making; conditions of democratic responsiveness and accountability; and polls and electoral outcomes. His research focuses on political information, public opinion, political identities, norms, and election campaigns, within North American and European countries. His methodological interests concern the analysis of longitudinal data (time series, panel, network, etc.) with secondary interests in Bayesian analysis and survey/lab experiment design. His work has been published in journals such as the American Journal of Political Science, British Journal of Political Science and Journal of Politics.
Guy D. Whitten is the Department Head and Cullen-McFadden Professor of Political Science in the Bush School of Government and Public Service at Texas A&M University. His primary research interests are comparative political economy, comparative public policy, and political methodology. Much of his research has involved cross-national comparative studies of industrial democratic nations. He has published a variety of manuscripts in peer-reviewed journals, including the American Journal of Political Science, Armed Forces and Society, the British Journal of Political Science, Electoral Studies, the Journal of Politics, and Political Behavior. Together with Christine Lipsmeyer and Andrew Philips, he recently published The Politics of Budgets: Getting a Piece of the Pie (Cambridge University Press). He is an active reviewer of manuscripts, and currently serves on the editorial boards of Political Analysis and Political Science Research and Methods.